Machine Learning to Enable Superfast EV Charging
Idaho National Laboratory: Using AI tech, an EV battery can be charged to 90% in 10 minutes without damage.
August 22, 2022
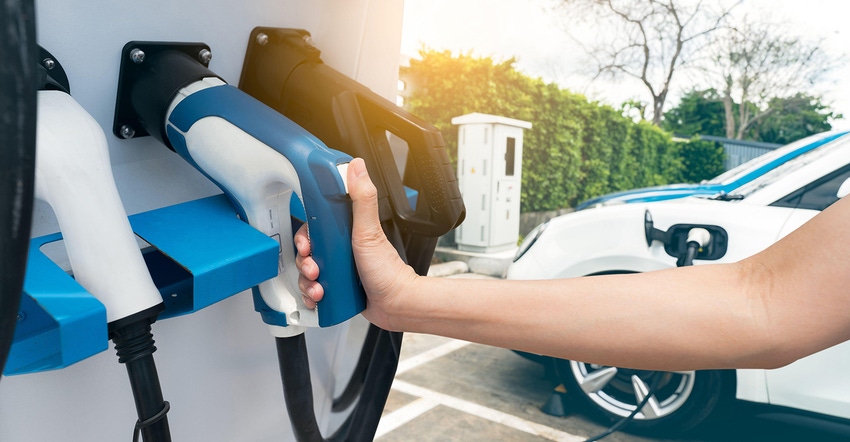
Despite the growing popularity of electric vehicles, many consumers still hesitate to make the switch. One reason is that it takes so much longer to power up an electric car than it does to gas up a conventional one. But speeding up the charging process can damage the battery and reduce its lifespan. Now, scientists report that they’ve designed superfast charging methods tailored to power different types of electric vehicle batteries in 10 minutes or less without harm.
The researchers were scheduled to present their results at the fall meeting of the American Chemical Society (ACS), according to an ACS news release. ACS Fall 2022 is a hybrid meeting being held virtually and in-person Aug. 21–25, with on-demand access available Aug. 26–Sept. 9. The meeting features nearly 11,000 presentations on a wide range of science topics.
“Fast charging is the key to increasing consumer confidence and overall adoption of electric vehicles,” stated Eric Dufek, Ph.D., who is presenting this work at the meeting. “It would allow vehicle charging to be very similar to filling up at a gas station.” Such an advance could help the U.S. reach President Biden’s goal that by 2030, half of all vehicles sold should be electric or hybrid.
Balancing Speed and Damage Risk
Charging the lithium-ion batteries that fuel electric vehicles is a delicate balancing act. Ideally, drivers want to power up as quickly as possible to get back on the highway, but with current technology, speeding up the process can cause damage. When a lithium-ion battery is being charged, lithium ions migrate from one side of the device, the cathode, to the other, the anode. By making the lithium ions migrate faster, the battery is charged more quickly, but sometimes the lithium ions don’t fully move into the anode. In this situation, lithium metal can build up, and this can trigger early battery failure. It can also cause the cathode to wear and crack. All of these issues will reduce the lifetime of the battery and the effective range of the vehicle — expensive and frustrating consequences for drivers.
One solution to this conundrum is to tailor the charging protocol in a way that optimizes speed while avoiding damage for the many different types of battery designs currently used in vehicles. But developing optimal protocols requires a huge amount of data on how various methods affect these devices’ lifetimes, efficiencies and safety. The design and condition of batteries, as well as the feasibility of applying a given charging protocol with the current electric grid infrastructure, are also key variables.
Machine Learning Steps In
To address these challenges, Dufek and his research team at Idaho National Laboratory now report the use of machine learning techniques that incorporate charging data to create unique charging protocols. By inputting information about the condition of many lithium-ion batteries during their charging and discharging cycles, the scientists trained the machine learning analysis to predict lifetimes and the ways that different designs would eventually fail. The team then fed that data back into the analysis to identify and optimize new protocols that they then tested on real batteries.
“We’ve significantly increased the amount of energy that can go into a battery cell in a short amount of time,” stated Dufek. “Currently, we’re seeing batteries charge to over 90% in 10 minutes without lithium plating or cathode cracking.”
Going from a nearly dead battery to one at 90% power in only 10 minutes is a far cry from current methods, which, at best, can get an electric vehicle to full charge in about half an hour. While many researchers are looking for methods to achieve this sort of super-fast charging, Dufek says that one advantage of their machine learning model is that it ties the protocols to the physics of what is actually happening in a battery.
Future Work
The researchers plan to use their model to develop even better methods and to help design new lithium-ion batteries that are optimized to undergo fast charging. Dufek stated that the ultimate goal is for electric vehicles to be able to “tell” charging stations how to power up their specific batteries quickly and safely.
The researchers acknowledge support and funding from the U.S. Department of Energy’s Vehicle Technologies Office.
Presentation Title: Extreme fast charging: Identification of failure modes and routes to improve performance
Abstract: Range anxiety is seen as a key limitation by many consumers looking to purchase an electric vehicle. The two routes to alleviate this anxiety are through the development of higher energy batteries and batteries capable of charging in 10 minutes or less. Achieving either target is difficult and presents a suite of challenges spanning from material degradation through cell and electrode design. When performing extreme fast charging, many types of degradation emerge including Li deposition and cathode cracking. Early detection and understanding using electrochemical methods are complicated, but possible if using a multitude of different signatures. Here we describe recent efforts to jointly align electrochemical methods with targeted characterization and advanced analysis to detect failure modes. Specifically, machine learning and other advanced analysis approaches show promise to reduce the time and effort needed to predict life, delineate failure modes, and provide input to electrochemical models. Here we discuss the use of machine learning to perform early failure mode classification on cells used for fast charge applications. Using this information, it is then possible to feedback information for the refinement of advanced charging protocols designed to minimize specific aging pathways.
About Idaho National Laboratory
Battelle Energy Alliance manages INL for the U.S. Department of Energy’s Office of Nuclear Energy. INL is the nation’s center for nuclear energy research and development, and also performs research in each of DOE’s strategic goal areas: energy, national security, science and the environment. For more information, visit www.inl.gov.
About the Author(s)
You May Also Like